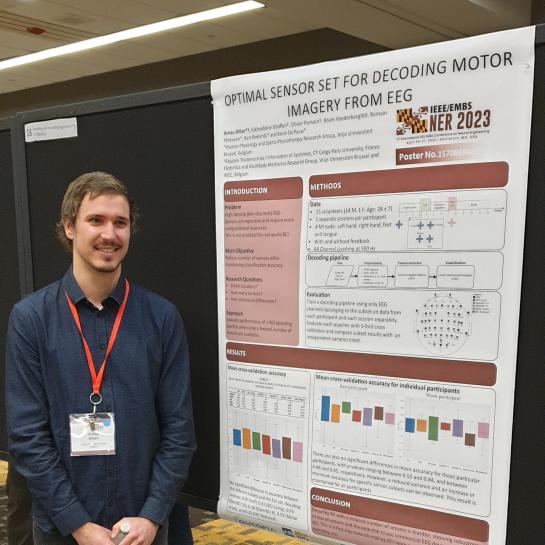
On April 26, 2023, Arnau Dillen presented our research on motor imagery decoding at the 11th International IEEE EMBS Conference on Neural Engineering in Baltimore with a poster presentation titled "Optimal sensor set for decoding motor imagery from EEG". (For social media #EEG #Motor imagery #BCI).
Brain-computer interfaces can be used to operate devices by detecting a person’s intention from their brain activity. Decoding motor imagery (MI) from electroencephalogram (EEG) signals is a commonly used approach for this purpose. To reliably identify MI from EEG signals, a sufficient number of sensors is usually required. However, a large number of sensors increases the computational cost of discriminating MI classes.
Furthermore, consumer-grade devices that measure EEG signals often employ a reduced number of sensors compared to medical- or research-grade devices. In this experimental study, we investigate the tradeoff between accuracy and complexity when decoding MI from a restricted number of EEG sensors. For this purpose, several decoding pipelines were trained on EEG data using different subsets of electrode locations employing well-established decoding methods.We found that there is no significant difference (p=[0.18–0.91]) in average decoding accuracy when using fewer sensors. The largest loss in performance for a single individual was a reduction in mean decoding accuracy of 0.1 when using 8 out of 64 available sensors. Decoding MI from a limited number of sensors is therefore feasible, highlighting the potential of using commercial sensor devices for this purpose to reduce both monetary and computational costs.